Crowd Counting Tech has Key Societal Impacts
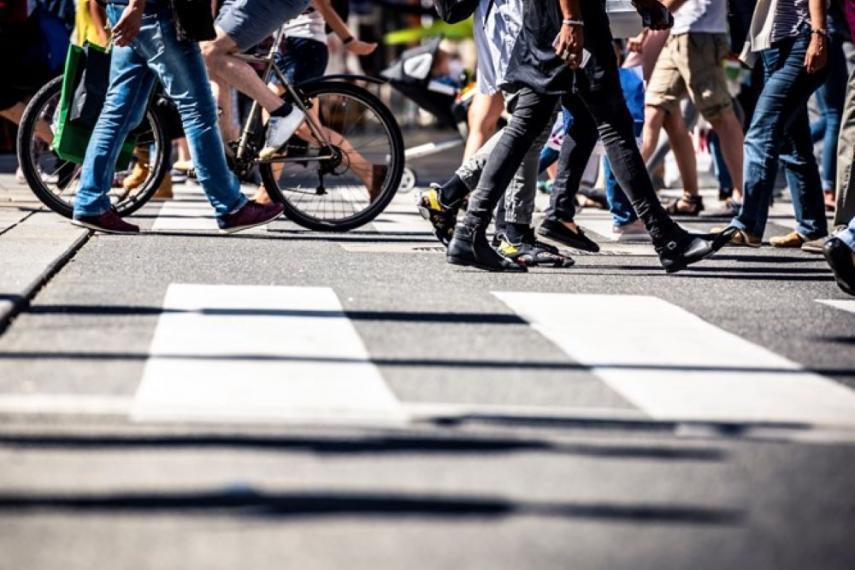
New computer vision methods for accurate crowd counting and informed decision making.
Traffic control. Public security. Social distancing enforcement. Precision agriculture.
These are just some of the societal applications made possible by crowd counting, a popular topic in computer vision. The field of computer vision studies how to use computers to extract information from images or videos. In crowd counting, computers can tally up the number of people, vehicles, or agricultural crop pests, for example. Since objects may be partially blocked and similar objects may appear to be different sizes due to perspective—a challenge known as scale variation—computers can struggle, resulting in low counting accuracy.
Dr. Minglun Gong, a professor and the director of the University of Guelph’s School of Computer Science, has worked with his PhD students to develop a novel Artificial Intelligence-based method to train computers to crowd count more effectively.
Like the Human Brain
For a computer to “see” and analyze an image, it learns from a neural network modelled loosely after the human brain. These networks are made up of many layers, which hold interconnected nodes that act like neurons in our brains—combining input signals and outputting the result. Gong’s team developed a novel neural network design that extracts features from multiple resolution scales. The result: the computer learns how to count objects with different scales and densities more accurately.
Testing the Model
The researchers evaluated their model by testing it on three existing crowd counting datasets and compared it to the best available crowd counting methods. They found that their model resulted in more accurate counts than existing methods, was more robust when image resolution was poor, and was more flexible—it could be trained using one dataset and applied to another, while maintaining its accuracy.
“Our new model addresses issues of scale variation, density shift, and overfitting,” says Gong. It will be a game changer for applications like video surveillance and traffic control. We are also applying the technique to social distance estimation for supporting pandemic control. We also plan to apply it to precision agriculture, such as crop yield estimation and pest control.”
Minglun Gong is a professor and the director of the School of Computer Science.
This work was supported by a Natural Sciences and Engineering Research Council of Canada Discovery Grant.
Wang M, Cai H, Zhou J, Gong M. Interlayer and intralayer scale aggregation for scale-invariant crowd counting. Neurocomputing. 2021 Jun 21. doi: 10.1016/j.neucom.2021.01.112.