Unmasking Fraud: How Smarter Algorithms Are Cracking Insurance Scams
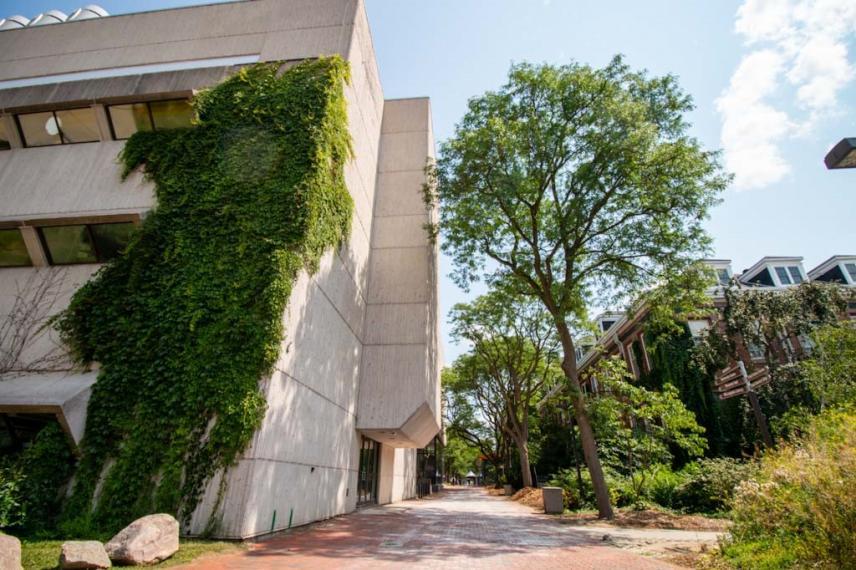
A new AI-powered method detects hidden fraud patterns with 91 per cent accuracy, offering a lifeline to insurers and policyholders.
In the shadowy world of insurance fraud, a single suspicious claim can cost companies millions, and honest customers higher premiums. But what if an algorithm could spot these scams before they spiral into costly outcomes for consumers?
A study by Francis Kwaku Combert and Dr. Anna T. Lawniczak in the University of Guelph’s Department of Mathematics and Statistics, along with Dr. Shengkun Xie, Toronto Metropolitan University, introduces a new tool: the BPW K-means clustering algorithm. This machine learning innovation does not just find fraud, it redefines how we uncover hidden patterns in oceans of data.
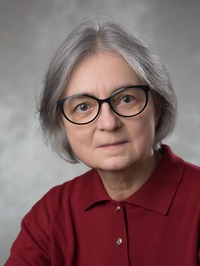
The Clustering Revolution
Traditional fraud detection typically relies on outdated methods that treat every piece of data as equally important. The new BPW K-means clustering algorithm acts like a high-tech magnet, a novel approach to this challenge. By splitting data features into two groups, critical and secondary, and weighting their importance, the algorithm pinpoints suspicious clusters with precision.
“Think of it as sorting groceries into two carts,” says Lawniczak.
“One cart holds the essentials you need immediately, like milk. The other has items you will use later. Our algorithm does this with data, prioritizing what matters most for catching fraud.”
The BPW K-means clustering algorithm focuses on the most telling clues of fraud while minimizing less critical details. It starts by splitting data features, like claim frequency or repair costs, into two groups: high-priority signals – such as how often claims are filed – and background details, such as geographic patterns. Then, it amplifies the key signals, giving them up to 90 percent influence in its analysis.
By clustering claims based on these weighted patterns, it groups suspicious activity, exposing fraud rings that traditional methods miss. Tested on real insurance data, it achieved 91 percent accuracy, outperforming older models by 38 per cent and even surpassing complex "black box" machine learning models. This proves that smarter prioritization, not complexity, is the key to unmasking hidden scams.
Why This Matters: Beyond Insurance
The BPW K-means clustering algorithm does not just solve a problem, it opens new possibilities. For insurers, it can pinpoint fraud hotspots, such as those identified in the study, helping decision-makers deploy investigators more efficiently.
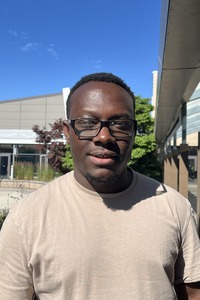
“This is not just about catching criminals,” says Mr. Combert. “It is about fairness. Every fraudulent claim avoided protects honest people from bearing the cost.”
The Bigger Picture
As fraud tactics evolve, so must our tools. The BPW K-means clustering algorithm bridges the gap between complex machine learning methods and real-world usability. For insurers, it shields against losses. For consumers, it is a step toward fairer premiums. For data scientists, it proves that sometimes, the smartest solutions are not the most complicated, just the most cleverly designed.
“In the fight against fraud, the smallest details, like a claim’s location or frequency, can be the loudest alarms,” says Dr. Lawniczak. “Our work improves discovering patterns hidden in the data.”
The work has additional applications beyond just cost efficiency in insurance claims. Beyond insurance, banks could use it to detect fraudulent transactions, while marketers might refine customer segmentation for sharper targeting.
Funding Acknowledgement: This work was supported by The Natural Sciences and Engineering Research Council of Canada (NSERC).
Combert, F.K.; Xie, S.; Lawniczak, A.T. Bi-Partitioned Feature-Weighted K-Means Clustering for Detecting Insurance Fraud Claim Patterns. Mathematics 2025, 13, 434. https://doi.org/10.3390/math13030434
This story was written by Mojtaba Safdari as part of the Science Communicators: Research @ CEPS initiative. Mojtaba is a PhD candidate in the School of Engineering under Dr. Amir A. Aliabadi.