AI Assists in Planning Radiation Treatments for Dogs
Brain tumours can develop in any dog breed at any age, but in 8-year-old dogs the chance of having a brain tumour is between 2.8-4.5 per cent. Cancer treatment is important to improving the longevity and quality of their lives. Radiation therapy exposes cancer cells to high doses of radiation over several weeks, fatally damaging their DNA, as one avenue.
Current radiation therapy planning relies on computed tomography (CT) scans to measure electron density, which effects how radiation travels and helps determine the correct radiation dose. Magnetic resonance imaging (MRI) scans provide detailed images of soft tissues for narrowly targeting the radiation site. MRI scans have better imaging quality, especially for tumours in areas where CT scans struggle to show clear differences between types of soft tissues, like in the brain. However, MRI scans do not provide electron density information, so they cannot be used alone in the radiation treatment planning process as the right dose of radiation cannot be calculated.
There has been significant interest in developing MRI-based radiation treatment plans, without using CT, through the use of machine learning and deep learning methods that can generate pseudo-CT images. These CT-like scans would be generated from the MRI imaging data. Aside from reducing radiation exposure, this approach could also reduce costs for dog owners and simplify treatment planning; to stay still enough for high-resolution MRI images, animals are typically sedated.
Researchers at the University of Guelph (U of G) explored the capabilities of a Conditional Generative Adversarial Network (CGAN) in generating pseudo-CT images that could assist veterinarians in diagnosing cancer and improving radiation treatment planning.
Can AI Generate Reliable Pseudo-CT Images?
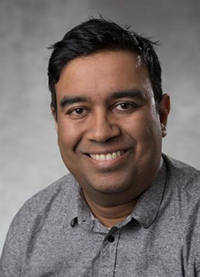
Dr. Eranga Ukwatta is an Associate Professor in U of G’s School of Engineering. His Guelph Imaging AI Lab has a key research focus in machine learning for medical imaging, to ultimately assist in veterinary clinical decision making.
A CGAN is a form of artificial intelligence (AI) that learns by generating and refining data through competition between two neural networks, and the output is tailored to specific conditions. Nicola Billings, a graduate student in Ukwatta’s Guelph Imaging Lab, trained a CGAN by feeding it pairs of MRI images and CT scans from canine OVC patients with brain tumours, where it then learned the relationship between the two imaging types. One neural network, the generator, then uses the MRI image data and attempts to create a realistic, pseudo-CT image. The other neural network, the discriminator, compares the generated CT image to real CT scans. If the pseudo-CT is too different, then the generator gets feedback to better refine its output pseudo-CT.
Since the CGAN was trained with real, paired data (real MRI and matching CT scans), the generator does not create random CT-like images, but rather it learns to generate CT scans that actually match anatomical structures in the given MRI image. The CGAN outputs were also evaluated by expert radiologists that assessed whether the generated CT images provided the necessary anatomical detail for diagnoses.
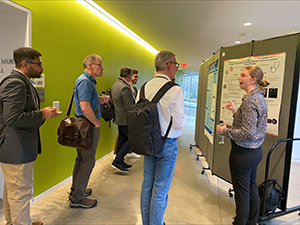
The Potential for AI-Assistance in Veterinary Diagnostics
The team’s findings suggest that pseudo-CT imaging has huge potential in veterinary medicine. If AI-generated CT images can offer equivalent diagnostic accuracy to real CT scans, veterinarians could use MRI data to produce CT-equivalent imaging without costly CT machines or exposing animals to unnecessary radiation and sedation. However, Billings notes that their investigation has limitations.
"While the models perform well, further improvements in image quality, training data and model refinement are necessary to reduce errors and enhance generalizability," says Ukwatta.
"Future studies should focus on optimizing the models for different dog breeds, validating evaluation scales, and exploring methods to reduce errors for improved clinical applicability in radiation therapy planning."
Billings N., Appleby R., Komeili A., Poirier V., Ukwatta E. Magnetic resonance imaging–based radiation treatment plans for dogs may be feasible with the use of generative adversarial networks. Am. J. Vet. Res. 2025. doi: 10.2460/ajvr.24.08.0248
This story was written by Jamie Dawson as part of the Science Communicators: Research @ CEPS initiative. Jamie is an M.Sc. candidate in the Chemistry Department under Dr. Mario A. Monteiro. Her research focus is on characterizing bacterial cell-surface carbohydrate structures to ultimately develop glycoconjugate vaccines.