Hack Attack
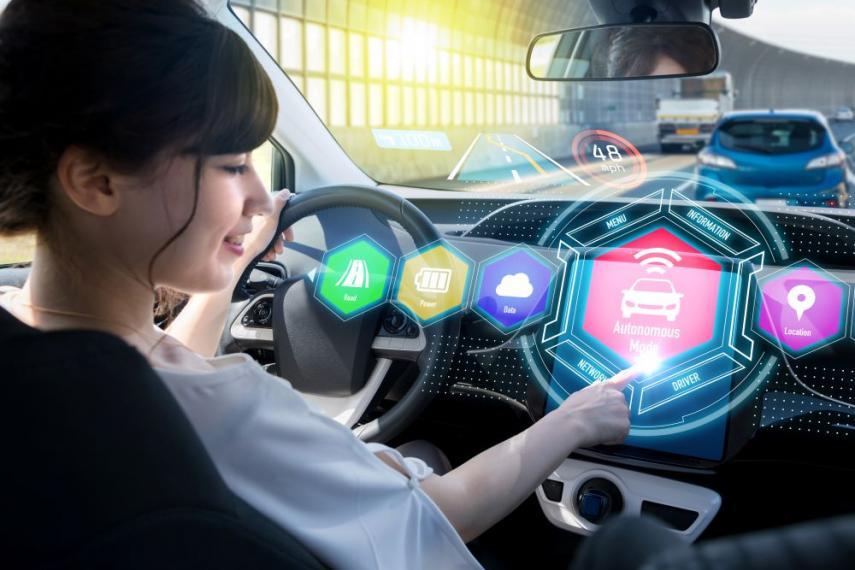
U of G researcher creates novel machine learning system to help prevent malicious software attacks.
We have all been there. Happily searching the Internet for that recipe that a co-worker recommended. A click on an innocent-looking website, and oh no! Up pops a nasty advertisement. In the days that follow, the computer is slow, and programs keep crashing, glitching, or functioning poorly. We were most likely a victim of malware, or malicious software. Malware is any software written with the intent of damaging devices, stealing data, and wreaking general havoc on networks and systems. Familiar examples include viruses, trojans, and ransomware.
Mitigating malware requires extensive resources and time, and unfortunately, attackers take advantage of our inability to efficiently detect and identify malware. In response to this challenge, computer science professor Ali Dehghantanha has collaborated with researchers from around the globe to develop a novel machine learning system that accurately identifies and differentiates between different types (families) of malware. This research is critical because malware is a key threat to essential technologies and networks. Malware is a particular risk for computer systems where physical and software components are linked and interact with each other, such as those used in newer and emerging technologies, like self-driving cars.
Existing machine learning systems used to detect malware struggle to classify and assign all malicious software to a malware family. However, most malware shares a high proportion of computer code, and the overlap in the code is key to identification. By implementing a novel algorithm, Dehghantana’s machine learning system identifies and analyzes the malware characteristics. The system finds overlap between codes, functionalities, and file properties, and categorizes the malware based on similarities with known families of malicious programs. The team sourced three malware datasets and evaluated the precision and accuracy of their system in classifying malware. For each of the three datasets, Dehghantanha’s system classified malware at an accuracy level that was consistently above 99 per cent.
“Our system identifies and analyzes malware efficiently and accurately, which will help mitigate potential damage to our critical infrastructure caused by malicious attackers,” explains Dehghantanha. “In future, we will evaluate our system against bigger datasets and improve its performance.”
This work was supported by the Department of National Defence, Innovation for Defence Excellence and Security, and the Natural Science and Engineering Research Council of Canada.
Alaeiyan M, Dehghantanha A, Dargahi T, Conti M, Parsa S. A multilabel fuzzy relevance clustering system for malware attack attribution in the edge layer of cyber-physical networks. ACM T Cyb-Phys Syst. 2020 Mar 12. doi: 10.1145/3351881.
To learn more, you can read Prof. Dehghantanha’s other work on this subject:
Darabian H, Dehghantanha A, Hashemi S, Homayoun S, Choo KK. An opcode‐based technique for polymorphic Internet of Things malware detection. Concurr Comp-Prac E. 2020 Mar 25. doi: 10.1002/cpe.5173.
Jahromi AN, Hashemi S, Dehghantanha A, Choo KK, Karimipour H, Newton DE, Parizi RM. An improved two-hidden-layer extreme learning machine for malware hunting. Comput Secur. 2020 Feb 1. doi: 10.1016/j.cose.2019.101655.
Dovom EM, Azmoodeh A, Dehghantanha A, Newton DE, Parizi RM, Karimipour H. Fuzzy pattern tree for edge malware detection and categorization in IoT. J Syst Architect. 2019 Aug 1. doi: 10.1016/j.sysarc.2019.01.017.
Jahromi AN, Hashemi S, Dehghantanha A, Parizi RM, Choo KK. An enhanced stacked lstm method with no random initialization for malware threat hunting in safety and time-critical systems. IEEE Trans Emerg Topics Comput Intell. 2020 Jun 22. doi: 10.1109/tetci.2019.2910243.