Statistical Tools Help Solve a Challenge in Biology
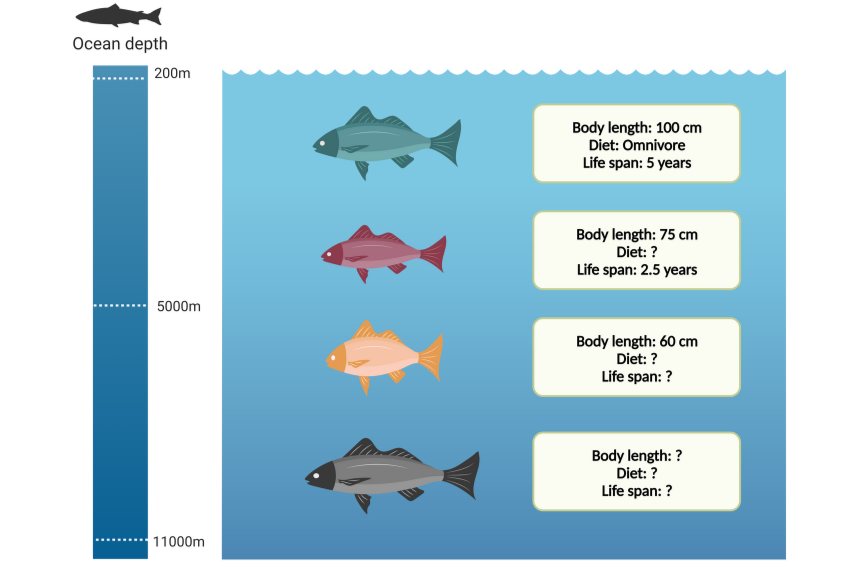
Researchers develop a strategy for analyzing incomplete data sets
Missing data is a common and challenging problem in a broad range of scientific studies. This is particularly true in the analyses of real-world data. For example, in the study of biological systems it is difficult to completely sample a population with a wide variety of traits (characteristics like body size, age and habitat). It is impossible to sample everything, and missing data is almost inevitable.
To add to the challenge, when data is missing, the missing pieces may not be completely random. For instance, species that live in less accessible regions of the world, such as deep oceans or remote areas, are more likely to have missing values in their trait data.
A common practice for handling missing data is to only analyze the “complete-case” data set, a subset of the data with no missing values. However, complete-case analysis discards a significant portion of data that can end up leading to a bias in the analysis and inaccurate conclusions.
Filling in the Missing Pieces
An alternative method for handling missing data is imputation, a statistical modeling approach that estimates missing values using the available data. Currently there is no single best method to impute data, as the accuracy of imputation models is highly dependent on the data in question. In addition, most of the available imputation models are validated based on simulated data sets, rather than real data.
Dr. Zeny Feng, a Professor in the Department of Mathematics & Statistics at the University of Guelph, proposed a method to identify the best tool for imputing missing values of real trait data. Dr. Jacqueline May, who recently defended her PhD in Bioinformatics, co-advised by Feng and Dr. Sarah Adamowicz from the Integrative Biology department, took up the challenge. Together, the team devised a new automated workflow to find the best imputation model for imputing missing trait data in a real data set.
They began by selecting nearly complete data sets from a set of real biological data and simulated missing values in these complete data sets. Different imputation methods were then used to impute the missing values and compared to the true observed trait values. The models were evaluated on their ability to accurately impute the missing data. The team also tested whether including information on the evolutionary history of the species improved the imputation accuracy. By comparing the accuracy of imputation among different methods, the best imputation method for the data could be selected.
A Strategy for Future Studies
This work provides a strategy for selecting the best method to use when imputing data in real studies. Each data set is unique, and the best method for one dataset may not be the best for the next. “It’s like having many gardening tools, but you’re not sure which one will be best,” says Feng. “You’d better test them before buying.”
Collaborating with biologists is something that motivates Feng. “I’m most excited about how statistical tools can be used to advance the field of biology,” she says. The problem of missing data extends beyond biology into other fields as well. Feng and her team will be testing their new workflow on more data sets with the hopes of helping scientists in other fields better analyze their results.
This story was written by Carley Miki as part of the Science Communicators: Research @ CEPS initiative. Miki is a PhD candidate in the Department of Physics under Dr. John Dutcher. Her research focus is on understanding the forces and interactions between soft, sugar-based nanoparticles and how they differ when charged.
Funding Acknowledgement: This works was supported by the Food from Thought: Agricultural Systems for a Healthy Planet Initiative program funded by the Government of Canada through the Canada First Research Excellence Fund, University of Guelph scholarships, Natural Sciences and Engineering Research Council of Canada (NSERC) Discovery grants, and by grants in Bioinformatics and Computational Biology funded by the Government of Canada through Genome Canada and Ontario Genomics and by the Ontario Ministry of Economic Development, Job Creation and Trade.
Reference: May, J. A., Feng, Z., Adamowicz, S. J. A real data-driven simulation strategy to select an imputation method for mixed-type trait data. PLoS Comput. Biol. 2023, 19(3): e1010154. doi: https://doi.org/10.1371/journal.pcbi.1010154