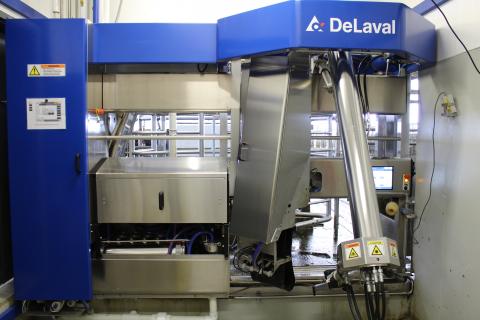
Robotic milking systems can lead to early disease detection in dairy herds.
By Sydney Pearce and Cate Willis
Analyzing thousands of robotic milking system records has taught University of Guelph dairy researchers a vital lesson—cow behavioural changes documented by such systems can help predict certain disease events earlier.
On-farm, these systems can let farmers know that cows are unwell up to four days or more before milk yields drop, allowing farmers to intervene sooner, says Dr. Trevor DeVries, a professor in the Ontario Agricultural College.
Meagan King, a former post-doctoral researcher in the Department of Animal Biosciences, and DeVries analyzed robotic milking system data from Ontario dairy farms to determine disease-indicating behaviours, such as declines in rumination behaviour and overall activity.
“Early disease detection allows producers to address illness in their herds earlier, improving both welfare and productivity on-farm,” says DeVries. “It can also improve animal welfare by enabling farmers to more effectively use preventive and therapeutic treatments that minimize animal discomfort.”
Researchers gathered data from more than 600 cows over eight months. They looked at behavioural changes in individuals that experienced illnesses such as displaced abomasum (also known as twisted stomach), lameness and mastitis, an inflammatory mammary gland infection.
Data was collected from behavioural monitoring devices resembling Fitbits on each cow and from the milking systems that the devices communicate with.
Cows could visit the automated milking systems (AMS) several times a day, providing a huge pool of data to work with. This mass of data allowed researchers to determine that lower activity and shorter rumination time can predict disease onset between one and two weeks earlier than visual diagnosis.
The researchers have since used these data to develop advanced, machine-learning mathematical models to precisely detect mastitis in dairy cows.
Detection models traditionally use fewer variables than are measured on AMS farms, DeVries says. This model is more precise because it integrates more variables that are regularly measured on robotic or automated milking farms.
“It was groundbreaking,” he says. “It showed that we can approach disease detection with these types of data and they will allow us to detect disease with more accuracy.”
Collaborators include Dr. Stephen Leblanc, a professor in the Department of Population Medicine in U of G’s Ontario Veterinary College; Drs. Ed Pajor, Syed Ali Naqvi and Herman Barkema from the University of Calgary; and Dr. Tom Wright, a dairy specialist with the Ontario Ministry of Agriculture, Food and Rural Affairs.
The research was funded by Dairy Farmers of Canada, the Canada First Research Excellence Fund and the Ontario Ministry of Agriculture, Food and Rural Affairs through the Ontario Agri-Food Innovation Alliance.