CARE-AI Seminar Series: Dr. Eran Tal (Virtual)
Date and Time
Location
Virtual
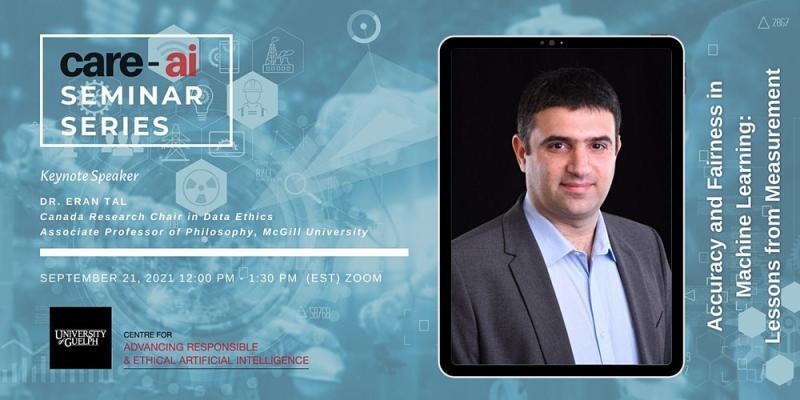
Details
The accuracy and fairness of machine learning models are often assumed to be separate, and to some extent conflicting, desiderata. I argue that this is a mistake that partially stems from an overly simplistic understanding of accuracy. Using insights from the philosophy of measurement, I distinguish among three sources of inaccuracy in supervised machine learning: label bias, modeling bias, and fitness bias. While the machine learning community has focused on minimizing label and modeling bias, it has tended to shift responsibility for fitness bias to users. I show that evaluating fitness bias is necessary for determining the predictive accuracy of machine learning models, and that doing so is a crucial step toward resolving many challenges that are currently classified exclusively under the ‘fairness’ heading.
Eran Tal is Canada Research Chair in Data Ethics and Associate Professor of Philosophy at McGill University. His work deals with the epistemic and ethical dimensions of data collection, data analysis, and data use in the sciences. He has contributed to the philosophy of measurement, an area within philosophy of science that deals with the concepts and problems involved in designing, operating, and interpreting measurement procedures. His current research projects concern the conceptual foundations of psychometrics, and the ethical and social implications of big data and machine learning algorithms.