Seeing Clearly
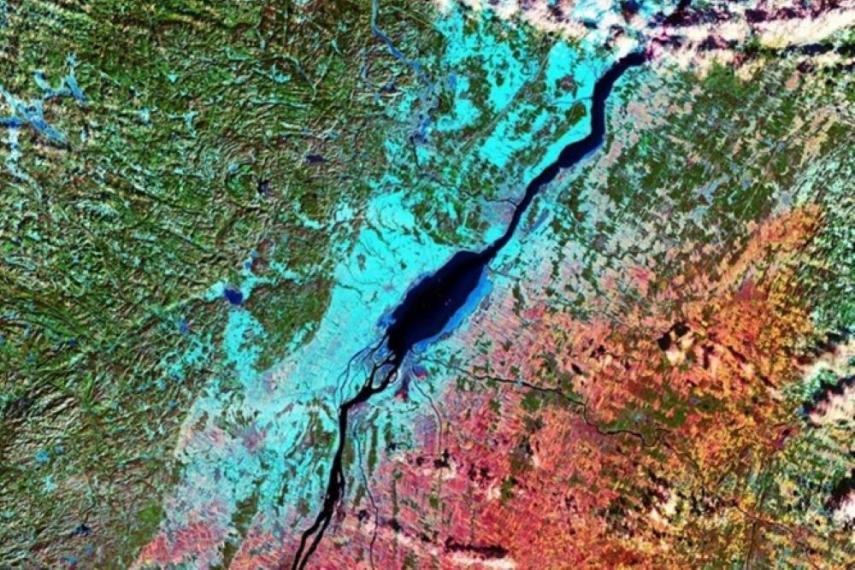
U of G engineers use machine learning to improve images of our planet.
Not just a pretty picture, remote sensing imagery has shed light on major world events, such as the Australian bushfires. These powerful images are generated using sensor technologies, usually carried by satellites that orbit the Earth. For example, NASA’s Landsat 8 takes approximately 500 snapshots of our planet’s surface each day. People then manually “classify” features, such as trees and rivers, that are captured by the snapshots. The process is labour-intensive, but machine learning can help.
University of Guelph engineering professors Graham Taylor and Shawki Areibi, along with PhD student Ahmed Elshamli sought to improve upon machine learning methods used to generate remote sensing images. The researchers tackled the “domain adaptation problem.” In domain adaptation, a computer learns how to identify a feature, like a tree, by scanning an image that has been classified by a human operator. The computer applies that learned information to classify other features. However, if the context changes, the system struggles—it may not recognize a tree that is covered in snow if it was trained using images from summer months. In those cases, domain adaptation systems need to be retrained with relevant images, making the process inefficient.
The U of G researchers developed a new domain adaptation technique that efficiently handles large-scale remote sensing datasets with minimal involvement from human researchers. Their “adaptive multisource domain adaptation” technique uses a simplified algorithm and data from different contexts to train the system. The researchers tested their technique using local climate zone (LCZ) data—landcover maps for urban landscapes—that were generated as part of a contest. The contest challenge was to take training data made available for five cities and use it to predict the local climate zone maps for four test cities. The U of G researchers deployed several domain adaptation techniques—including the new one—on this data.
“Our adaptive multisource domain adaptation method consistently improved on baseline training methods,” says Dr. Taylor. “With this new technique, we will be able to analyze enormous datasets more efficiently. In future, we plan to explore the performance and scalability of this technique by conducting an even larger LCZ experiment.”
Graham Taylor holds a Tier 2 Canada Research Chair in Machine Learning.
Elshamli A, Taylor GW, Areibi S. Multisource domain adaptation for remote sensing using deep neural networks. IEEE Trans Geosci Remote Sens. 2019 Dec 17. doi: 10.1109/TGRS.2019.2953328