CARE-AI Awards $60K to Innovative AI Projects
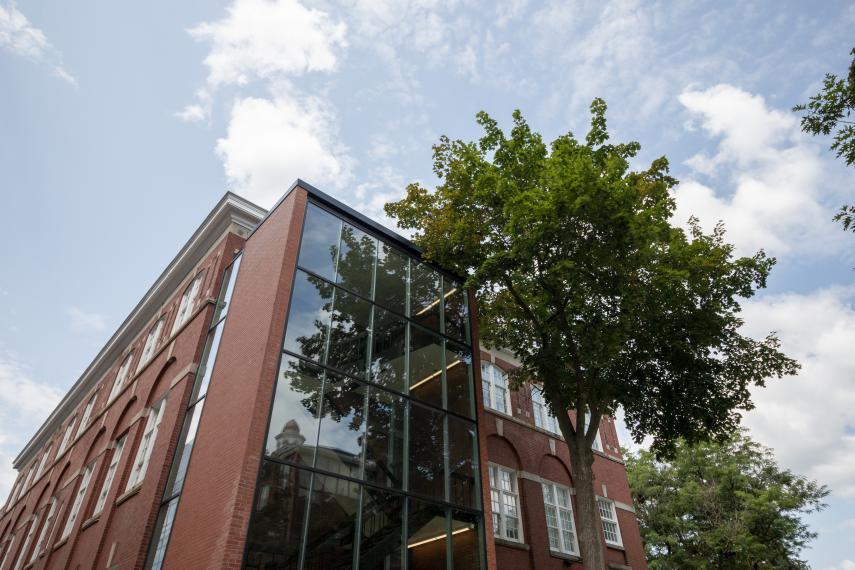
U of G’s AI centre awards seed funding to projects spanning environment, health and societal wellness.
Environmental concerns with flood radar and pollutants, risk modelling management in finance, and automated health predictions are a glimpse of the research areas that will be advanced by University of Guelph (U of G) artificial intelligence experts, resulting, in part, from the latest Centre for Advancing Responsible and Ethical Artificial Intelligence (CARE-AI) seed funding.
U of G’s CARE-AI seeks to advance multidisciplinary AI training and research alongside responsible application, evidenced by the latest projects emerging from its funding round.
“The range of projects showcase the breadth of AI and its growing role across virtually every sector,” says Kevin Matsui, managing director for CARE-AI. “In funding these multidisciplinary research projects, CARE-AI is able to help advance more ethical and responsible use of AI in a range of areas that are critical to improving society.”
Improving Environmental Monitoring
Drs. Petros Spachos and Erica Pensini from the School of Engineering aim to reduce the costly and time-consuming process of water quality analysis for pollutants. Traditionally, the process involves manual sample collection from field sites, shipping and analysis in a lab and data processing.
The research team will employ deep learning methods through each of the three steps in the process from data collection to analysis, using new processing hardware and wireless technologies. Their ultimate goal is to build an intelligent system that can perform real-time water pollution monitoring and pollution prediction
Dr. Stefan Kremer in the School of Computer Science, Dr. Genevieve Ali in the School of Environmental Sciences (Ontario Agricultural College) and PhD student Jason Kemp will use AI to help keep homeowners and properties safe by improving our ability to predict flooding.
Their research will move beyond traditional ground-based data used in flood estimation, which relies on climate measurements like precipitation or wind, in favour of a new approach: Using machine learning models to extract information from radar images. This will ultimately enable earlier streamflow predictions, provide better predictions in remote regions with limited ground-based data and ensure adequate time is available for homeowners to prepare for predicted floods.
Automating Healthcare Diagnostics
Dr. Eranga Ukwatta, School of Engineering and Dr. Richa Mittal, radiologist with Guelph General Hospital, aim to develop automated survival rate predictions of five-year lung cancer patients, using Computed Tomography (CT) scans.
The research team will use CT images and develop a deep learning model for tumour detection and segmentation. Images of tumours will be taken and analyzed over a period time to monitor their progression and regression. Prognostication, the likelihood of the development or progression of a disease, will be performed using a deep learning-based imaging biomarker. The intended result is a standardized method for quantifying mortality risk, enabling more efficient decision-making for physicians and even earlier tumour detection.
Dr. Ryan Appleby in the Department of Clinical Studies (Ontario Veterinary College), as well as Drs. Amin Komeili and Eranga Ukwatta in the School of Engineering, will use AI to improve radiographic image quality in canine images, reducing costs and radiation exposures associated with repeated radiograph images.
Radiographs, such as X-rays, are common tools in pet health diagnostics, but there can be errors, such as mispositioning the patient or inappropriate exposure when the image is taken. The research team will leverage deep learning methods, including convolutional neural networks, to develop an algorithm that will assess the radiograph quality and its appropriateness for veterinarians and support staff.
AI for Better Societal Wellbeing
Dr. Fattane Zarrinkalam in the School of Engineering and Dr. Zeinab Noorian at Toronto Metropolitan University are tackling the challenge of social media misinformation by developing trust models to determine the credibility of information sources in social media content.
In the age of misinformation, the project’s goal is to assess and recommend more trustworthy sources of content to users, such as with basic COVID-19 information. They propose to build a computational trust model that will harvest social data and evaluate its contextual credibility, which could be integrated into social media algorithms. Ultimately, the model would provide early detection of organized and systematic efforts to disseminate misinformation.
Dr. Nikola Gradojevic with the Department of Economics and Finance (Gordon S. Lang School of Business and Economics) and Dragan Kukoj with Serbia-based RT RK Institute for Computer Based Systems will use a new machine learning framework to enhance model risk management in business finance.
The research team will employ a machine learning non-linear model and fuzzy logic controller (FLC), a type of mathematical system. The machine learning model risk indicators, including statistical, profitability, network and unintended bias measures, will serve as inputs to the FLC. This will result in a framework to help model risk management with a focus on protected groups and financial crises, such as COVID-19 economic impacts and unintended biases in AI models.