Secure and Intelligent: Protecting Our Connected Data
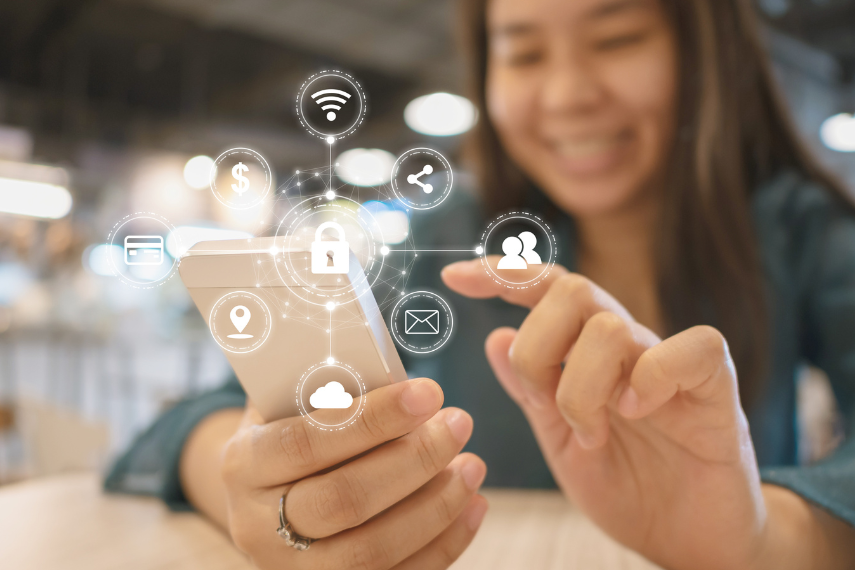
Smart Devices, Big Risks
In an era where smart devices pervade every corner of our lives, securing the vast data they generate against misuse and theft is more crucial than ever. This narrative is not just about technology, but about preserving our privacy and safety in a world increasingly reliant on the Consumer Internet of Things (CIoT). The expanding network of CIoT devices, from smart refrigerators to wearable fitness trackers, holds the promise of a more connected and efficient life. It also opens the door to security vulnerabilities and privacy concerns. This is a world where every data from your daily life has the potential to be exploited. Indeed, the scenario underscores the importance of developing robust security frameworks that can defend against such threats, turning the promise of a smart, interconnected world into a safe reality for everyone.
Navigating the digital landscape of CIoT presents a series of intricate challenges that go beyond the typical cybersecurity concerns. The primary issue lies in the sheer volume and sensitivity of data collected by CIoT devices, which, if compromised, could lead to severe privacy violations and financial losses. Additionally, the decentralized nature of these devices introduces complexities in data management and security protocol implementation, making them susceptible to sophisticated cyber-attacks, including data leakage and model inference attacks. These challenges underscore the necessity for innovative solutions capable of safeguarding our digital ecosystem while maintaining the functional integrity and efficiency of CIoT devices.
Innovative Privacy Preserving Solution for Digital Minefield
The solution to keeping our smart devices safe involves a clever combination of teamwork and secret-keeping techniques, proposed by Drs. Ali Dehghantanha, Abbas Yazdinejad and their research team. The model involves a two-level privacy-preserving framework that synergizes Federated Learning and Partially Homomorphic Encryption (PHE). Think of Federated Learning as a team of smart devices working together to learn and improve without sending their data out of the house. They share their learning outcomes, not the data itself. To add an extra layer of privacy, PHE is a mechanism that allows these outcomes to be mixed and compared without ever revealing the actual secrets they contain. This innovative approach ensures that data remains encrypted throughout the learning process, enabling computations on encrypted data without revealing sensitive information. Meanwhile, it offers a robust defence against potential data breaches and attacks. This solution not only secures data transmission between devices and the central server but also maintains the integrity and confidentiality of the data, marking a significant step forward in addressing the vulnerabilities inherent in CIoT environments.
From Theory to Reality: Safety First
To implement and test the model, the approach was hands-on and practical. The team set up a secure environment where smart devices could learn and share insights without risking privacy. This approach has been shown to enhance the detection of security threats and reduce false alarms, making our smart devices not just smarter but also safer by protecting against potential hacks or data breaches.
"Our work is fundamentally about enhancing user privacy and security within the realm of the CIoT,” says Abbas Yazdinejad. By leveraging advanced encryption techniques and federated learning, we aim to establish a robust framework that significantly elevates the privacy and security standards of CIoT devices. This research underscores the importance of protecting user data in a world increasingly dominated by smart technologies, marking a critical step towards safer and more secure digital environments".
This story was written by Kasra Ghasemi as part of the Science Communicators: Research @ CEPS initiative. Ghasemi is a PhD candidate in the School of Engineering under Drs. Syeda Humaira Tasnim and Shohel Mahmud.
Reference: E. Rabieinejad, A. Yazdinejad, A. Dehghantanha, and G. Srivastava, “Two-Level Privacy Preserving Framework: Federated Learning for Attack Detection in the Consumer Internet of Things,” IEEE Trans. Consum. Electron., Jan. 2024, doi: 10.1109/TCE.2024.3349490.