CIFAR Azrieli Global Scholar Profile: How Graham Taylor champions AI innovation & entrepreneurship
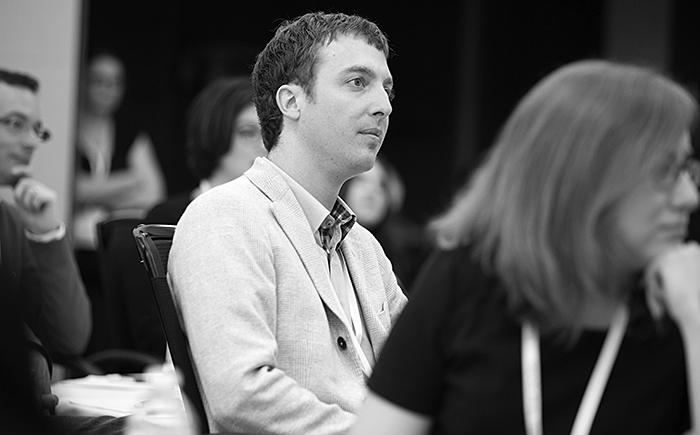
When 20 teams gathered for NextAI’s first venture day this September, competing to become the next Shopify, Uber or Wealthsimple – but powered by the machine learning technology this incubator hopes to help commercialize – the big winner was a machine-learning powered garbage bin that sorts and diverts waste away from landfills, while extracting consumption and demographic insights. It may seem odd, but over the past few years AI has spread ever more widely into our daily lives. Anticipating its next application could not only change the way we live our lives, but our entire economy.
“When I talk to people about AI and machine learning, I tell them I am excited for this technology because it has the potential to impact every sector,” says Graham Taylor, a CIFAR Azrieli Global Scholar and the academic director of NextAI’s incubator program. In this role Taylor oversaw the application of machine learning to fields as varied as finance, health, natural resources, human resources and waste management.
“The analogy is software. It’s virtually impossible to think of an industry that hasn’t been transformed by software. Machine learning is, in essence, software writing software. And while it’s a bit scary to think of it that way, you realize that the opportunities are endless.”
NextAI is just one of the AI initiatives Taylor, a machine learning expert and professor at the University of Guelph’s School of Engineering, has a hand in. From CIFAR’s Learning in Machines & Brains program, to the newly created Vector Institute, to his connections to the Creative Destruction Lab and his role as scientific advisor to a handful of machine learning start-ups, Taylor’s is deeply involved in Canada’s AI leadership.
Of course, all this would not be possible had it not been for his original brush with the field as a fourth-year systems design engineering student at the University of Waterloo. His professor had the students build their own AIs to play the board game Abalone and ran the course like a showdown. The teams were instructed to incorporate some form of learning into their AIs rather than have them be completely rules based. Taylor’s team put a lot of work into the project and won. He was hooked.
Now, after studying under AI visionaries like Geoffrey Hinton and Yann Lecun, he tries his best to share his expertise on as many projects as possible. Prior to signing up with NextAI, Taylor was a co-founder at Kindred, a start-up that focuses on using robots to move warehouse inventory and recently won a contract with Gap. He remains a scientific advisor to the prospering start-up but has funneled more of his energies into NextAI where he has been able to teach aspiring entrepreneurs machine learning and help them build companies, find funding and customers, build up their products and navigate the start-up world.
“Even though I was teaching the machine learning course, I was learning so much,” says Taylor of the experience.
This year, Taylor also joined the newly formed Vector Institute in Toronto as a faculty member. Vector’s mission is to attract global AI talent to Canada and work with academic institutions, industry, start-ups, incubators and accelerators to advance AI research and drive the application, adoption and commercialization of AI technologies here. One of Taylor’s responsibilities with the organization is to engage with entrepreneurship programs like NextAI’s incubator.
“For many years, Canada lost its graduates to private R&D facilities in the USA because these labs simply did not exist in this country. In the past year, we’ve seen RBC Research, Google Brain, Google Deep Mind, Facebook AI Research, Uber, Microsoft, and Samsung open machine learning-focused research labs in Canada. It’s incredible,” says Taylor.
Taylor’s extensive involvement in the AI space relies heavily on his research expertise. At Guelph he runs one of the largest labs at the School of Engineering. His 20 students and staff are tackling a number of themes and projects from the more abstract to pure application, including agricultural models for targeted spraying to improve yields and decrease environmental impact.
One of the more abstract problems Taylor is working on is dataset augmentation, the process of taking existing data, and slightly transforming it in order to create larger data sets. For image sets this might involve zooming in on a picture of say a dog, cropping it, rotating it and adding some noise or grain. Then you see if the algorithm can still identify it as a dog.
Teaching neural networks to learning from limited data is a key step in expanding AI applications to more and more fields. When it comes to images of dogs, we have more than enough examples to feed an algorithm, but in other domains we lack the amount of data current algorithms need. Ultimately, some AI researchers would like to see algorithms learn like humans – from just a few or even just one example.
Taylor’s team does not have human-level learning in mind with their work but they do want to figure out how to augment data in a way that can be universally applied to any type of data, not just visual.
“When you test an algorithm on data it hasn’t seen before, you would expect it to see colour changes and scale changes and lighting changes,” says Taylor. In other domains we don’t really know how to create all these transformations because we don’t understand the data as well.”
To address this problem, Taylor’s team is transforming data in a different way. First they simplify the data and map it onto a new layer of representation. (In neural networks, each layer the data becomes more abstract.) In these abstract layers, whether data originated as an image or a piece of audio is not discernable. It is at this abstract layer that Taylor and his team manipulate the data. They then input it back into the data set, making it larger and giving the algorithm more to work with.
Another project they’ve taken on is training an algorithm to know what it knows. AI experts have approached this problem in different ways in the past showing that an algorithm learns faster when it learns according to a human-designed curriculum, which exposes the algorithm to data in a specific order and at a specific pace – easy examples first, then progressively harder. Self-paced algorithms would do the same but without the human-designed curriculum. One way to train a system to be aware of it’s own knowledge is to penalize it for a wrong answer, but not for not answering or asking for a hint. This way, it learns to represent its confidence in solving certain tasks and researchers learn what kinds of tasks it struggles with. Often, these tasks are the ones that are ambiguous to us as well.
Taylor and his team are trying to connect the confidence measure aspect of self-aware algorithms to the self-paced learning scenario where the algorithm learns to develop its own curriculum. Combining these two features will make the algorithm faster and more accurate. Having algorithms that can learn faster, from fewer examples, and still maintain accuracy greatly increases the potential applications of this technology.
But with the spread of AI comes a growing concern for societal impact. How is Taylor dealing with the increased public interest in how this technology will impact our lives?
When it comes to ethics, he credits CIFAR for changing the way he thinks about his role in the discussion.
“CIFAR has completely changed my perspective on this. Before CIFAR if someone asked me about ethics I would generally say, I’m a technology researcher, I do the tech part, I don’t know anything about ethics, I don’t know if I’m qualified to even comment on this. Now, I think it’s important as a researcher to at least talk about it.”
But rather than addressing concerns about bias, fairness, and privacy using only legislation or regulation, he is a proponent of using technology or algorithms themselves to improve fairness. Other fellows in CIFAR’s Learning in Machines & Brains program, such as Richard Zemel, are already working on such solutions and in Taylor’s lab, they’re exploring the issue of explainability – whether an algorithm can explain its decision – something that could help us gauge whether its actions are biased or not.
Taylor recounts a quote from a fellow advisor in the NextAI program, Kathryn Hume of Integrate AI. “Even though we like to think of algorithms as object, they tend to magnify our own human biases, because they’re trained from data sets that are collected from human judgements.”
With that in mind, Taylor will be part of a group of researchers advising the Canadian government on how to navigate the rising tide of disruptive technologies like AI this November, with an aim to finding a balance between bolstering Canada’s economy and insuring that all corners of society benefit.
“Not only will machine learning write software much more effectively and efficiently than we do not, it will write program that are currently out of reach for humans,” says Taylor. “These surprises are just around the corner, and we as Canadians will be proud, because much of it will happen here in this country.”