New research applies machine learning to better predict stock market volatility
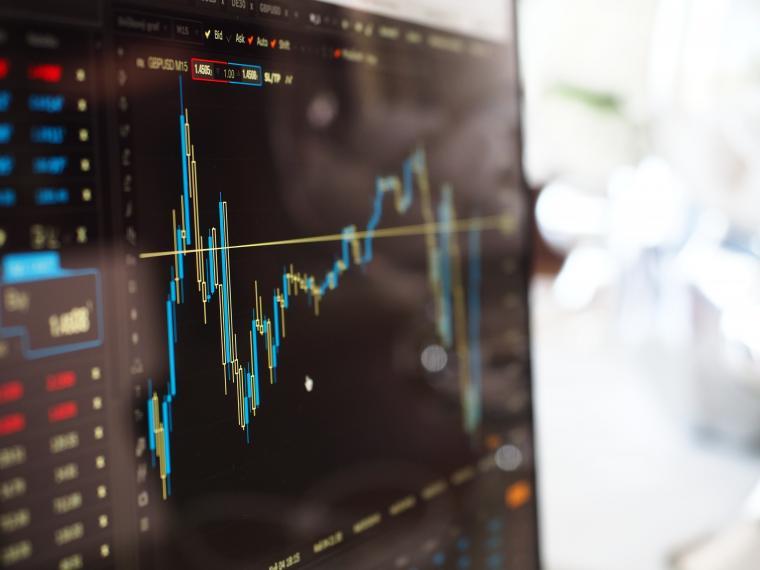
Financial crises, such as the Great Recession, have revealed the importance of understanding stock market volatility, the most widely used risk measure, which tracks the degree of fluctuation in stock prices. “Accurate and interpretable volatility forecasts can aid risk management and guide regulatory actions aimed at preventing or mitigating financial crises,” explains Dr. Fred Liu, Assistant Professor of Finance.
Utilizing a much larger number of predictor variables than previously possible, Dr. Liu is employing artificial intelligence in his SSHRC Insight Development grant-funded project.
“Using Machine Learning and Big Data to Improve the Accuracy and Interpretability of Volatility Forecasts.” Machine learning substantially broadens the traditional approach, which was limited to a small number of variables, by incorporating hundreds of new variables for analysis.
Conventional models in economics and finance utilized a limited number of predictor variables, primarily historical prices. In contrast, machine learning has increased the number of variables into the hundreds, including macroeconomic variables, firm-specific variables, and variables derived from using textual data, high-frequency stock data, and options data. “This project's primary innovation is its capacity to utilize an immense number of variables from diverse data sources. These variables can be grouped into categories such as price trends, firm fundamentals, liquidity, and risk variables,” explains Dr. Liu.
Dr. Liu's research was inspired by conversations with financial professionals and regulators in various countries. He recalls, "I engaged in discussions with regulators from the Federal Reserve Bank, Bank of Canada, and European Central Bank. These exchanges helped me identify the information they deemed valuable in achieving financial stability objectives." Dr. Liu noted that Canadian bank risk managers seek machine-learning models that provide greater accuracy and interpretability than existing models. He emphasizes that while the financial industry prioritizes accuracy, regulators are more concerned with interpretability.
Dr. Liu gathers data for his project from publicly available sources, such as the Federal Reserve Economic Database, and plans to share his code with regulators and the financial industry. By granting broad access to this code, Dr. Liu believes a variety of financial professionals will be better equipped to manage risks. "Deeper insights into volatility forecasting can prove to be highly valuable in portfolio management, empowering professionals to better manage their portfolios. From a regulatory standpoint, it offers a clearer understanding of the factors driving volatility, potentially enabling proactive action."
The research is a natural continuation of Dr. Liu’s past research, which focused on risk management. “I am deeply passionate about designing and developing machine learning models for risk management, as I believe they can benefit society as a whole.”
Dr. Liu also takes pride in preparing the next generation of machine learning practitioners and researchers, especially considering the field's rapid evolution in recent years. "This project offers a fantastic opportunity for my undergraduate and graduate research associates to gain hands-on experience with the latest machine learning tools in Python, as well as financial and economic domain knowledge in this area. This expertise is highly valuable for both industry employment and the academic job market. Moreover, the insights from this project will be incorporated into case studies for my undergraduate and graduate financial machine learning courses."